Institute for Computational Biomedicine
AG Univ. Prof. Andreas Schuppert
The focus of Schuppert Group ranges from hybrid modelling and next generation computational technologies up to quantum computing for applications in medicine and health care. The group’s goal is to integrate knowledge- and physics-inspired models with AI technologies to create digital twins that help realizing model training in data sparsity and gain predictive models that satisfy the needs of clinical use. Current application areas include intensive care, pain research, rare diseases and oncology. Throughout the SARS-CoV-2 pandemics, the Schuppert group contributed to the nationwide modelling activities with a focus on simulating the peak load for intensive care capacities. These activities are followed up together with partners in MONID and NUM.
Projects
- SimLab Digital Patient is part of the NHR4CES consortium.
The Simlab will foster High Performance Computing for Medical applications. We strive to set up a HPC platform for the development and application of digital patient models for research and clinics.
https://www.nhr4ces.de/index.php/support/
- HDS-LEE - Helmholtz graduate school for Data Science – Life, Earth and Energy intends to develop new mathematical methods and computational technologies for a broad range of applications. Within HDS-LEE we develop hybrid technologies to learn the structure of poorly understood biological mechanisms, e.g. drug response, from data.
https://www.hds-lee.de
- ASIC: Algorithmic Surveillance of ICU patients
The demand for intensive care medicine will strongly increase over the next years facing unmet medical needs, such as early diagnosis of the acute respiratory distress syndrome (ARDS). Within the ASIC project we are developing a system for continuous analysis of data obtained from the hospital patient data management system (PDMS) in order to enable model-based ‘algorithmic surveillance’ of the state of critically ill patients. We are developing and integrating a hybrid modeling system which consists of two components: the Virtual Patient (VP) and the Diagnostic Expert Advisor (DEA). The VP is a model-based system which relies on the physiological models of respiratory and cardiac system and allows personalized modeling of a patient physiology. In contrast to the VP the DEA is a data-driven component which utilizes ML tools and will support the VP model in stratification of patients and parameters estimation for individual patients. These two components together build up a hybrid modeling system which will enable individual prognosis for a particular patient. ASIC is one of the use cases of the BMBF-funded consortium SMITH.
https://www.medizininformatik-initiative.de/de/asic-algorithmische-ueberwachung-der-intensivversorgung - Hybrid Modelling
Integration of prior knowledge into machine learning technologies in order to reduce the data demand for training, to foster the reliability of the models and to enable extrapolation, plays a crucial role for digitalization of medicine. Based on our prior work on structured hybrid models integrating process structures and machine learning in chemical engineering, we focus our research on the special mathematical challenges arising from the integration of medical data structures as well as the structures of medical knowledge into machine learning. Our research projects are dedicated to clinical applications characterized by heterogeneous, multi-scale structures in close cooperation with clinical and non-clinical research partners.
- Prognosis – MONID BMBF
The BMBF-funded project PROGNOSIS, which is part of the Germany-wide modelling hub MONID, aims to develop an integrated platform for simulation of epidemic and pandemic infection dynamics and its consequences for the healthcare systems on heterogeneous levels of care up to hospital logistics. Our group contributes models for prediction of loads on various levels of hospital care dedicated to precise simulations around peak loads. Moreover we contribute to the conceptual design of mixed data-driven and mechanistic modellng approaches for prediction of infection dynamics covering the challenges arising from the apparent lacks in data and quantitative understanding of mechanisms as well as the short predition horizons of pure data driven models.
- Bio2integrate
Integration of data from wearable devices (smart watch) and clinical data in order to improve the pain management for patients suffering from small fiber neuropathy. To read more about this work click here.
- TREAT-SGS
Schinzel-Giedion Syndrome (SGS) is a rare disease that affects young children. One debilitating symptom of SGS are frequent seizures that are difficult to manage using conventional therapies. In this consortium project we conduct a preclinical study to repurpose a drug to treat the seizures associated with Schinzel-Giedion-Syndrome. The project is funded by European Joint Programme on Rare Diseases via the Deutsche Forschungsgemeinschaft (DFG).
EDITH
The EU-funded EDITH consortium starting October 2022 strives to develop a roadmap for development and implementation of Digital Twins for Health Care. The roadmap will address the broad range of challenges to be tackled en route to digital twins from regulatory issues, high performance computing, computational technologies integrating AI and Systems Medicine up to clinical data. Use cases will demonstrate the route towards realisation of the potential of digital twins in health care.- CCLS
Started as an initiative by the Uniklinik RWTH Aachen and RWTH Aachen University, the Center for Computational Life Sciences (CCLS) constitutes a contact point and competence center between the disciplines of life sciences, medicine and computational sciences. Its main goal is to foster interdisciplinary research, teaching and infrastructure at the interface of life science and and cutting-edge information technology. In that context, the CCLS organises a colloquium, regular seminars and conferences on the one hand and enhances data availability on the other hand.
Andreas Schuppert
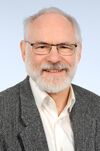
Andreas Schuppert is Professor for Computational Biomedicine and founding director of the Joint Research Center for Computational Biomedicine at RWTH Aachen University. He studied Physics and got a PhD in mathematics from University Stuttgart, followed by research and development positions in chemical-pharmaceutical industry. In 2007 he became Adjunct Professor at RWTH Aachen, where he became founding director of the Joint Research Center for Computational Biomedicine in 2013, a private-public partnership between RWTH Aachen University Hospital Aachen and Bayer AG. Since 2017 he is head of the Institute for Computational Biomedicine. His focus is on research and development of hybrid modelling technologies with focus on applications in intensive care, oncology, pain research. During the Covid19 pandemics he developed the DIVI prognosis tool and focused on pattern recognition in pandemic dynamics.
Hülya Ulu-Esser
Administrative Assistant
Tel.: 0241 80-85890
Fax: 0241 80-3385890
huluukaachende
Navid Akbari Alashti
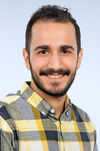
Navid Alashti joined the group in 2021 as a student researcher during his master’s studies in Simulation Sciences. He contributed to the modularization of a prognosis tool developed by Andreas Schuppert and completed his master's thesis focused on forecasting the number of age-stratified Covid-19 infections in German states using LSTM networks.
In 2023, he started his work as a PhD candidate, concentrating on the DISTANCE project, where he aims to develop models that predict post intensive care syndromes by monitoring health features of patients that are released from the ICU. He is also a member of the prognosis project and works on the identification of trend switches by comparing long- and short-term predictions.
Moein Einollahzadeh Samadi
Moein Samadi is a doctoral candidate in Computational Engineering Science at RWTH Aachen University and an associated Ph.D. student at the Helmholtz School for Data Science in Life, Earth, and Energy (HDS-LEE). Moein's doctoral research focuses on Design of experiments for the applications of mechanistic/data-driven hybrid models in life sciences. He develops methods and pipelines for incorporating clinical and biological mechanistic knowledge into conventional machine learning methods with the goal of improving the explainability, predictivity, and reliability of AI in medicine.
OrcidiD
Jorge Guzman Maldonado
Jorge Guzman Maldonado is a PhD student in Schuppert’s group and part of the HDS-LEE graduate school (Helmholtz School for Data Science in Life, Earth and Energy). He is working on the application of hybrid models, a combination of mechanistic modelling and machine learning techniques, to the reconstruction of signalling networks from drug response data. His background is in physics and mathematical engineering (UACH, Mexico) and he has a master in mathematical modelling (L’Aquila, Italy and Hamburg, Germany) with focus on complex systems. His research interests lie in the field of systems biology, particularly network modelling and inverse problems.
Marc-Daniel Hagel

Marc-Daniel Hagel began studying biology at the RWTH Aachen focusing mainly on molecular medicine, but he, later on, switched his focus toward computational biology and machine learning. As a PhD candidate, he is now working on mutation-driven developmental perturbations of gene expression in the SGS Project. The goal of this project is to find the underlying cause for the increased neuronal excitability which results in painful epileptic seizures in children suffering from this developmental disease. Therefore Marc-Daniel Hagel's focus lies on investigating differences in gene expression, genetic networks, and changes in brain development to find the driving forces as well as suitable therapeutic access points.
Researchgate
Jonas Kupschus
Jonas Kupschus is a PhD student at the Institute for Computational Biomedicine and part of the NHR graduate school (Alliance for National High Performance Computing). His research focuses on digital patients in the context of neuropathic pain. He is working on data driven modeling on large-scale patient data, as well as mechanistic models in the form of dynamical neuron models. Working closely with clinicians at Aachen University Hospital, his interest lies in personalizing general knowledge gained from large-scale patient data to more accurately fit individual patients and deepen the understanding of pain patients’ highly individual disease expression.
Micha Landoll
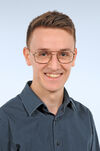
Micha Landoll is a research associate and PhD candidate in the interdisciplinary field of medicine, medical engineering, and computational biomedicine. His research focuses on in-silico clinical trials for cardiopulmonary therapies. Currently, he is working at the JRC on the PROGNOSIS project, a joint project to predict the spread of COVID-19 and the bed load of intensive care units in Germany. Additionally, he is affiliated with the Institute of Applied Medical Engineering at Uniklinik RWTH and the ARDS and ECMO Centre in Cologne-Merheim.
Joana Elena Meyer
Joana Meyer is a PhD student with a double Bachelor's degree in Materials Science and Mechanical Engineering (Saarland University, Germany, and Oregon State University, USA) and a Master's degree in Simulation Science (RWTH Aachen, Germany). She joined the team in 2020 to work on her master's thesis on a machine learning approach to discover patterns in myeloid Malignancies using clinical data. Now, as part of HDS-LEE (Helmholtz School for Data Science in Life, Earth and Energy), she is researching hybrid models that integrate adaptive control AI and knowledge-based components for predictive simulation in medicine. The goal is to demonstrate the algorithm using Covid19 pandemic data or critical care patient data and evaluate the impact of new control measures on outcomes.
Younes Müller
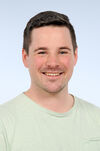
Younes Müller studied Computer Science at the RWTH Aachen. After graduating he became a research assistant at the JRC, where he worked as student researcher before. There he helped to develop and publish HybridML, a software platform for generating and training hybrid models, using TensorFlow and Casadi. Using HybridML he created a hybrid machine learning model to investigate the relationship between socio-economic covariates and COVID-19 spreading in German federal states. He works in the PROGNOSIS project, a joint project of 5 German Universities, aiming to predict COVID-19 spreading and ICU usage in Germany. He is in charge of the CMDA software, a small, lightweight tool to preprocess time series and generate features for machine learning, developed by Jorge Guzman.
ResearchGate
ScienceDirect
Kateryna Nikulina
Kateryna Nikulina is a research assistant at the Institute for Computational Biomedicine. She has a Master's degree in Accountancy and Audit (Kyiv, Ukraine), Bachelor's degree in Computer Science (RWTH Aachen) and now obtains the second Master's degree in Data Science (RWTH Aachen). Kateryna has joined the institute for ASIC project as a student assistant in 2020. As part of the project, she studied methods data preproccessing and comparison of populations from different origins, including convex hull analysis and classical machine learning methods. Kateryna successfully completed her Bachelor's thesis at the institute. Now, she is writing her Master's thesis on the topic of virtual patients, combining mechanistic models and machine learning tools for medical prediction improvement.
Richard Polzin
As a PhD student in the Institute for Computational Biomedicine Richard is currently working on the prediction of lung failure in intensive care units. After obtaining his bachelor's degree at FH Aachen, as well as a master's degree in Maastricht he joined the team of Prof. Schuppert in 2018.
While studying in Maastricht his focus was on machine learning and natural language processing. The project he's currently working on aims to improve patient care, especially with respect to acute respiratory distress syndrome, in the ICU. Thus regular measurements for various parameters are utilized and the techniques applied focus on time-series modelling and prediction.
Github
ResearchGate
Shukti Ramkiran
Shukti Ramkiran serves as a part-time manager at the Center for Computational Life Sciences (CCLS) while pursuing a PhD in Computational Psychiatry at the RWTH Aachen. Within the CCLS, she plays a pivotal role in managing key organizational tasks and contributes to academic teaching. Her research primarily centers on the development and application of computational methodologies to analyze neuroimaging data, with the aim of enhancing the understanding of psychiatric disorders.
Lisa-Katrin Schätzle
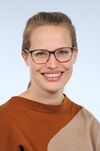
After finishing her PhD in the group of Andreas Schuppert in November 2020, Lisa Schätzle is continuing her research as a postdoctoral fellow. Her thesis focused on the systematic modeling of drug efficacy in cancer patients, including the development of the R-package FORESEE. Her main focus currently lies on her role as scientific coordinator of the Center for Computational Life Sciences.
OrcidiD
Jonas Wolber
Jonas Wolber is a PhD student in the group of Prof. Schuppert. At the same time he is also part of the Institute for Digitalisation and General Medicine at RWTH Aachen. As part of his PhD, he is monitoring patients with diabetes type 2 and metabolic dysfunction-associated steatotic liver disease using wearable devices. The aim of the study is to find out lifestyle patterns that can characterize those patients. His work also includes finding new innovative approaches to analyze health monitoring data of patients using large language models and machine learning. As part of two working groups his PhD work sits at the bridge between computational research and patient care.
Visiting Scientists
Dr. Jeyashree Krishnan
After completing her PhD with Andreas Schuppert in 2019, Jeyashree Krishnan now works as a data science consultant at Siemens AG and continues her research as a visiting researcher at the Joint Research Center for Computational Biomedicine. Previously her work focussed on modeling phase transitions in biological networks and in gaining a systems-level understanding of neurodegenerative diseases using machine learning models. Her current work expands on these topics with additional contributions to the area of digital twins as part of the EDITH project.
Google Scholar
Alumni
Dr. Konstantin Sharafutdinov
Dr. Patrick Stumpf
Dr. Kilian Merkelbach
Jayesh Sudhir Bhat
Dr. Marc Brehme
Maximilian Eck
Pejman Farhadi Ghalati
Dr. Ali Hadizadeh Esfahani
Dr. Jeyashree Krishnan
Dr. Nina Kusch
Dr. Michael Lenz
Dr. Maryam Montazeri
Dr. Satya Swarup Samal
A. Original papers
- A.Schuppert (1989): Error estimates and stability of ultrasound tomography reconstructions. In: Model Optimization in Exploration Geophysics, vol. 3 (A.Vogel, ed.). Vieweg, Braunschweig.
- A.Schuppert (1993): A model for the creep of oriented high-modulus fibers. Macromol. Chem.Theory Simul. 2, 643-651.
- N.Nefedov, K.Schneider, A.Schuppert (1994): Jumping behavior in singularly perturbed systems modelling bimolecular reactions. Weierstrass Institute for Analysis and Stochastics (WIAS) preprint No. 137, ISSN 0946-8633.
- S.Miesbach, A.Schuppert (1995): Neuronale Netze zur Analyse und Optimierung chemischer Produktionsprozesse. Der Maschinenmarkt 101, 40-43.
- A.Schuppert (1995): Mathematik in der Modellierung am Beispiel der mechanischen Eigenschaften von polymeren Hochleistungsfasern. In: Mathematik in der Praxis - Fallstudien aus Industrie, Wirtschaft, Naturwissenschaften und Medizin (A.Bachem, M.Jünger, R.Schrader, eds.). Springer, Heidelberg. 137-150.
- A.Schuppert (1995): Modelling the influence of monomer properties on hydrogen bond density in oriented aramid copolymers. J.Chem.Soc.Faraday Trans. 91, 2629-2631.
- N.Nefedov, K.Schneider, A.Schuppert (1996): Jumping behavior of the reaction rate of fast bimolecular reactions. Z.Angew.Math.Mech. 76, 69-72.
- M.Efendiev, B.Fiedler, A.Schuppert (1996): Upscaling of some exothermic reactions from the chemical industry. Preprint 15/96 of the Special Programme "Dynamics, analysis, efficient simulation and ergodic theory" of the German Federal Research Foundation (DFG). Z.Angew.Math.Mech. 76, 153-156.
- B.Fiedler, A.Schuppert, M.Efendiev (1996): Thermally stable upscaling of a model exothermic reactor. Preprint 38/96 of the Special Programme "Dynamics, analysis, efficient simulation and ergodic theory" of the DFG.
- A.Schuppert (1996): Modelling reaction kinetics - an interdisciplinary challenge for mathematics. In: Progress in Industrial Mathematics (H.Neunzert, ed.). Wiley & Teubner, Stuttgart. 159-165.
- A.Schuppert (1996): New approaches to data-oriented reaction modelling. Proceedings of the 3rd Workshop on Modelling of Chemical Reaction Systems (IWR, Heidelberg).
- M.Efendiev, A.Schuppert, M.Wolfrum (1997): Upscaling of chemical reactors: Comparison of mathematical and experimental results. In: Proceedings on Analysis, Numerics, and Applications. Addison Wesley Longman.
- M.Efendiev, M.Wolfrum, A.Schuppert (1997): Isothermal upscaling of chemical reactors: Two examples. Nonlin.Anal.Theory Meth.Appl. 30, 3455-3461.
- B.Fiedler, A.Schuppert, M.Efendiev (1997): Thermisch stabiles Upscaling einer exothermen Modellreaktion. In: Mathematik: Schlüsseltechnologie für die Zukunft (K.H.Hoffmann, W.Jaeger, T.Lohmann, H.Schunck, eds.), Springer, Heidelberg, 83-90.
- M.Bruns, J.Baurmeister, T.Schaefer, and A.Schuppert (1997): Mit Simulation Reserven mobilisieren. Nachr.Chem.Tech.Lab. 45, 483-485.
- G.Mogk, A.Schuppert (1998): Dynamische Modellierung und Analyse chemischer Reaktionssysteme. Technical Report Federal Research Ministry (BMBF) 03 D0022C
- A.Schuppert (1998): Prozessmodellierung mit Hybridmodellen. Chem.Ing.Tech. 70, 1090-1091.
- A.Schuppert (1999): Extrapolability of structured hybrid models: A key to the optimization of complex processes. In: Proceedings of the International Conference on Differential Equations (Equadiff) (B.Fiedler, K.Groeger, J.Sprekels, eds.) World Scientific Publishing, 1135-1151.
- M.A.Efendiev, A.Schuppert (1999). Stability analysis of chemical reactors. In: Scientific Computing in Chemical Engineering II (F,Keil, ed.) Springer, 225-230.
- R.Perne, A.Schuppert (2000): Advanced modelling and simulation technologies - The key challenges for process optimization. In: Proceedings of AspenWorld 2000, Orlando/FL (on CD).
- M.A.Efendiev, G.Habermehl, A.Schuppert (2000): On the stability of complex chemical reactors: an example, In: VW-Stiftung Proceedings (H.Schulze, ed.), Cottbus, 1-12.
- R.Perne, A.Schuppert (2002): Process Software in the Chemical Industry – the Challenge of Complexity. Comp.Aid.Chem.Eng. 10, 23-30.
- G.Mogk, Th.Mrziglod, A.Schuppert (2002): Application of Hybrid Models in the Chemical Industry. Comp.Aid.Chem.Eng. 10, 931-936.
- B.Fiedler, M.Efendiev, L.Lerman, J.Rademacher, A.Schuppert (2003): Stability analysis of reactors from the chemical industry. In: Mathematics – Key Technology for the Future, Springer Berlin (W.Jäger, ed.), 175-183.
- S.Rommel, A.Schuppert (2004): Data Mining for Bioprocess Optimization. Eng.Life Sci. 4, 266-270.
- A.Ohrenberg, C.v.Törne, A.Schuppert, B.Knab (2005): Application of Data Mining and Evolutionary Optimization in catalyst discovery and high-throughput experimentation – techniques, strategies, software. QSAR & Comb.Sci. 24, 29-37.
- A.Schuppert, R.Perne (2005): Data Mining mit Prozessdaten. Automatisierungstechnik, 53, 342-349.
- G.Schopfer, O.Kahrs, W.Marquardt, M.Warncke, T.Mrziglod, A.Schuppert (2005): Semi-empirical process modelling with integration of commercial modelling tools, In: Proceedings of ESCAPE-15, Elsevier (L.Puigjaner, A.Espuña, eds.), 595-600.
- B.Fiedler, A.Schuppert (2008): Local identification of scalar hybrid models with tree structure, IMA Journal of Applied Mathematics, 73, 449-476.
- S.Schneckener, L.Görlitz, L., H.Ellinger-Ziegelbauer, H.-J. Ahr, A Schuppert (2010): An elastic network theory to identify characteristic stress response genes, Comp.Biol.Chem. 34, 193-202.
- R.Ummanni, F.Mundt, H.Pospisil, S.Venz, C.Scharf, C.Barett, M.Fälth, J.Köllermann, R.Walther, T.Schlomm, G.Sauter, C.Bokemeyer, H.Sültmann, A.Schuppert, T.H.Brümmendorf, S.Balabanov (2011): Identification of clinically relevant protein targets in prostate cancer with 2D-DIGE coupled mass spectrometry and systems biology network platform. PLoS One. Feb 11;6(2):e16833.
- A.Schuppert (2011): Efficient reengineering of meso-scale topologies for functional networks in biomedical applications. J.Math.Ind. 1:6 doi:10.1186/2190-5983-1-6.
- S.Schneckener, N.Arden, A.Schuppert, (2011): Quantifying stability in gene list ranking across microarray derived clinical biomarkers, BMC Med.Genom. 4:73 doi:10.1186/1755-8794-4-73.
- F.J.Müller, A.Schuppert, (2011): Few inputs can reprogram biological networks, Nature 478, doi:10.1038/nature10543.
- B.M.Schuldt, F.J.Müller, A.Schuppert, (2012): What can networks do for you? In: New frontiers of network analysis in Systems Biology. (A.Ma'ayan, B.D.MacArthur, eds.) Springer, 173-194.
- A.Schuppert. (2012): Mathematics in Health Care (invited Feature). Newsletter of the European Mathematical Society, 83, 29-36.
- B.D.MacArthur, A.Sevilla, M.Lenz, F.J.Müller, B.Schuldt, A.Schuppert, S.J.Ridden, M.Fidalgo, J.Wang, I.R.Lemischka (2012): nanog-dependent feedback loops maintain alternate states of stem cell pluripotency, Nature Cell Biology, (doi:10.1038/ncb2603).
- Schuldt BM, Guhr A, Lenz M, Kobold S, MacArthur BD, et al. (2013): Power-Laws and the Use of Pluripotent Stem Cell Lines. PLoS ONE 8(1): e52068. doi:10.1371/journal.pone.0052068.
- M. Krauss, R. Burghaus, J. Lippert, M. Niemi, P. Neuvonen, A. Schuppert, St. Willmann, L. Kuepfer, L. Görlitz (2013):Using Bayesian-PBPK modeling for assessment of inter-individual variability and subgroup stratification, In Silico Pharmacology, 1:6 doi:10.1186/2193-9616-1-6.
- Schaller S, Willmann S, Lippert J, Schaupp L, Pieber T, Schuppert A, Eissing T. (2013): A Generic Integrated Physiologically based Whole-body Model of the Glucose-Insulin-Glucagon Regulatory System. CPT: Pharmacometrics & Systems Pharmacology 2, e65; doi:10.1038/psp.2013.40.
- Lenz M., Schuldt B.-M., MüllerF.-J., Schuppert, A.(2013): PhysioSpace: Relating gene expression experiments from heterogeneous sources using shared physiological processes PLoS ONE 01/2013; 8(10):e77627.
- Balabanov S, Wilhelm T, Venz S, Keller G, Scharf C, Pospisil H, Braig M, Barett C, Bokemeyer C, Walther R, Brümmendorf TH, Schuppert A, Combination of a proteomics approach and reengineering of meso scale network models for prediction of mode-of-action for tyrosine kinase inhibitors PLoS One [2013, 8(1):e53668] PMID:23326482 PMCID:PMC3541187.
- Braig M., Pällmann N., Preukschas M., Steinemann D., Hofmann W., Gompf A., Streichert T., Braunschweig T., Copland M., Rudolph K L., Bokemeyer C., Koschmieder S., Schuppert A., Balabanov S, Brümmendorf T H (2014): A 'telomere-associated secretory phenotype' (TASP) cooperates with BCR-ABL to drive malignant proliferation of leukemic cells. Leukemia, doi: 10.1038/leu.2014.95.
- Wolkenhauer O., Auffray Ch., Brass O., Clairambault J., Deutsch A., Drasdo D., Gervasio F., Preziosi L., Maini Ph., Marciniak-Czochra A., Kossow Ch., Kuepfer L., Rateitschak K., Ramis-Conde I., Ribba B., Schuppert A., Smallwood R., Stamatakos G., Winter F., Byrne H. (2014): Enabling multiscale modeling in systems medicine, Genome Medicine, 6:21.
- Lenz M, Goetzke R, Schenk A, Schubert C, Veeck J, Hemeda H, Koschmieder S, Zenke M, Schuppert A, Wagner W. (2015): Epigenetic biomarker to support classification into pluripotent and non-pluripotent cells. Sci Rep. Volume 5, p. 8973.
- Wagener R, Lenz M, Schuldt B, Lenz I, Schuppert A, Siebert R, Müller FJ. (2015): Investigation of potential traces of pluripotency in germinal-center-derived B-cell lymphomas driven by MYC. Blood Cancer J Volume 5 (2015) p. e317.
- Schaller S, Lippert J, Schaupp L, Pieber T, Schuppert A, Eissing T (2015): Robust PBPK/PD based Model Predictive Control of Blood Glucose. IEEE Trans Biomed Eng. 2015 Nov 2. [Epub ahead of print].
- Krauss M, Tappe K, Schuppert A, Kuepfer L, Goerlitz L (2015): Bayesian Population Physiologically-Based Pharmacokinetic (PBPK) Approach for a Physiologically Realistic Characterization of Interindividual Variability in Clinically Relevant Populations, PLoS ONE 10(10):e0139423.
- Kuepfer L, Schuppert A., Systems Medicine in Pharmaceutical Research and Development. Methods Mol Biol, Volume 1386 (2016), p. 87-104.
- Lenz M, Müller FJ, Zenke M, Schuppert A, Principal components analysis and the reported low intrinsic dimensionality of gene expression microarray data. Sci Rep [2016, 6:25696] PMID:27254731 PMCID:PMC4890592.
- Brehme M, Koschmieder S, Montazeri M, Copland M, Oehler VG, Radich JP, Brümmendorf TH, Schuppert A, Combined Population Dynamics and Entropy Modelling Supports Patient Stratification in Chronic Myeloid Leukemia. Sci Rep [2016, 6:24057] PMID:27254731 PMCID:PMC4890592.
- Krauss M, Hofmann U, Schafmayer C, Igel S, Schlender J, Mueller C, Brosch M, Schoenfels W, Erhart W, Schuppert A, Block M, Schaeffeler E, Boehmer G, Goerlitz L, Hoecker J, Lippert J, Kerb R, Hampe J, Kuepfer L, Schwab M (2017) Translational learning from clinical studies predicts drug pharmacokinetics across patient populations. npj Systems Biology and Applications 3(1): p. 11.
- Stumpf P. S., Smith R.C.G., Lenz M., Schuppert A., Müller F.-J., Babtie A., Chan Th. E., Stumpf M.P.H., Please C. P., Howison S. D., Arai F. , MacArthur B.D., (2017) Stem Cell Differentiation as a Non-Markov Stochastic Process, Cell Systems , Volume 5 , Issue 3 , 268 - 282.e7.
- Arne Schenk, Ahmed Ghallab, Ute Hofmann, Reham Hassan, Michael Schwarz, Andreas Schuppert, Lars Ole Schwen, Albert Braeuning, Donato Teutonico, Jan G. Hengstler & Lars Kuepfer (2017) Physiologically-based modelling in mice suggests an aggravated loss of clearance capacity after toxic liver damage, Scientific Reports 7, doi:10.1038/s41598-017-04574-z.
- Ehsani A., Niedenfuehr S., Eissing Th., Behnken S., Schuppert A. (2017) How to Use Mechanistic Metabolic Modeling to Ensure High Quality Glycoprotein Production, Computer Aided Chemical Engineering, Vol. 40, 2839-2844.
- Reiss L K, Schuppert A, Uhlig, St. (2018) Inflammatory processes during acute respiratory distress syndrome: a complex system, Current Opinion in Critical Care: February 2018 - Volume 24 - Issue 1 - p 1–9.
- Esfahani A., Sverchkova A., Saez-Rodriguez J., Schuppert A., Brehme M. (2018) A systematic atlas of chaperome deregulation topologies across the human cancer landscape, PLoS Comp. Biology, doi.org/10.1371/journal.pcbi.1005890.
- Apweiler Rolf, Beissbarth Tim, Berthold Michael R, Blüthgen Nils, Burmeister Yvonne, Dammann Olaf, Deutsch Andreas, Feuerhake Friedrich, Franke Andre, Hasenauer Jan, Hoffmann Steve, Höfer Thomas, Jansen Peter LM, Kaderali Lars, Klingmüller Ursula, Koch Ina, Kohlbacher Oliver, Kuepfer Lars, Lammert Frank Maier Dieter, Pfeifer Nico Radde Nicole, Rehm Markus, Roeder Ingo, Saez-Rodriguez Julio, Sax Ulrich, Schmeck Bernd, Schuppert Andreas, Seilheimer Bernd, Theis Fabian, Vera Julio, Wolkenhauer, Olaf (2018) Whither Systems Medicine? Experimental & Molecular Medicine vol. 50, page e453 (2018) doi:10.1038/emm.2017.290.
- Schuppert A., Mrziglod Th., (2018) Hybrid Model Identification and Discrimination with Practical Examples from the Chemical Industry, in: Hybrid Modelling in Process Industries, Jarka Glassey & Moritz v. Stosch eds., CRC Press, p63-88.
- Turnhoff L., Kusch N., Schuppert A. (2018) “Big Data and Dynamics” – The mathematical toolkit towards personalized medicine, in: Patterns of Dynamics, Gurevich P., Hell J., Sandstede B., Scheel A., Springer Proceedings in Mathematics & Statistics, p. 338-370.
- Winter, A. et al., (2018) Smart Medical Information Technology for Healthcare (SMITH), Methods Inf Med 2018; 57(S 01): e92-e105.
- H. Fröhlich, R. Balling, N. Beerenwinkel,, O. Kohlbacher, S. Kumar, Th. Lengauerm M.H. Maathuis, Y. Moreau, S.A. Murphy, T.M. Przytycka, M. Rebhan, H. Röst, A. Schuppert, M. Schwab, R. Spang, D. Stekhoven, J. Sun, A. Weber, D. Ziemenk and B. Zupan (2018) From hype to reality: data science enabling personalized medicine, BMC Medicine, 2018, 16:150.
- Ch. Müller, F. Weysser, Th. Mrziglod, A. Schuppert (2018) Markov-Chain Monte-Carlo Methods and non-identifiabilities, Monte Carlo Methods and Applications, Vol. 24, 3, DOI: https://doi.org/10.1515/mcma-2018-0018.
- Boulier, F., Fages, F., Radolescu, O., Samal, S., Schuppert, A., Seiler W., Sturm Th., Walcher S., Weber, A. (2019) The SYMBIONT project: symbolic methods for biological networks, ACM Communications in Computer Algebra, Volume 52 Issue 3, September 2018 Pages 67-70.
- Turnhoff L, Hadizadeh Esfahani A, Montazeri M, Kusch N, Schuppert A. (2019) FORESEE: a tool for the systematic comparison of translational drug response modeling pipelines, Bioinformatics, Volume 35, Issue 19, 1 October 2019, Pages 3846–3848, https://doi.org/10.1093/bioinformatics/btz145.
- Farhadi-Galati, P., Samal, S.S., Bhat J.S., Deisz R., Marx G., Schuppert A. (2019) Critical Transitions in Intensive Care Units: A Sepsis Use Case, Scientific Reports volume 9, Article number: 12888 (2019).
- Stalmann, U., Ticcioni, F., Li, R., Wong ,A., Cowley, G., Root, D.E., Heckl, D., Snoeren, I., Martinez, S., Brümmendorf, T.B., Schuppert, A., Costa, I., Ebert, B., Schneider, R., (2019) Deconstructing the Clonal Advantage and Clonal Stability of 5q- Candidate Genes in Del(5q) MDS on a Single Cell Level Blood (2019) 134 (Supplement_1): 559., https://doi.org/10.1182/blood-2019-122588.
- Kusch N., Turnhoff, L., Schuppert A., (2019) Modeling from Molecule to Disease and Personalized Medicine, in: Handbook of Biomarkers and precision Medicine, C. Carini, M. Fidock, A. van Gool eds., CRC Press, pp 245-250.
- Kuepfer, L. Schuppert A. (2019) Systems Biology Approaches to Identify new Biomarkers, in: Handbook of Biomarkers and precision Medicine, C. Carini, M. Fidock, A. van Gool eds., CRC Press, pp 143-148.
- Baumeister, J., Chatain, N., Hubrich, A., Maie’ T., Costa I.G., Denecke, B., Han L., Küstermann C., Sontag S., Sere’ K., Strathmann K., Zenke M., Schuppert A., Brümmendorf, T. H., Kranc, K. R., Koschmieder S., Gezer, D., (2020) Hypoxia-inducible factor 1 (HIF-1) is a new therapeutic target in JAK2V617F-positive myeloproliferative neoplasms, Leukemia, 14.11.2019, https://doi.org/10.1038/s41375-019-0629-z.
- Ehsani, A., Kappatou, Ch.D., Mhamdi A., Mitsos A., Schuppert A. Niedenfuehr, S., (2019) Towards Model-Based Optimization for Quality by Design in Biotherapeutics Production, Computer Aided Chemical Engineering, Volume 46, 2019, Pages 25-30.
- Schätzle, L.K., Esfahani, A. H., Schuppert A., Methodological Challenges in Translational Drug Response Modeling in Cancer, PLoS Computational Biology, (2020) https://doi.org/10.1371/journal.pcbi.1007803.
- Krishnan, J., Torabi, R., Di Napoli, E., Schuppert A., (2020) A Modified Ising Model of Barabási-Albert Network with Gene-type Spin, Journal of Mathematical Biology volume 81, pages769–798(2020).
- Chrysoula Dimitra Kappatou, Alireza Ehsani, Sebastian Niedenführ, Adel Mhamdi, Andreas Schuppert, Alexander Mitsos, Quality-targeting dynamic optimization of monoclonal antibody production, Computers & Chemical Engineering, Volume 142, 2020, 107004, ISSN 0098-1354, doi.org/10.1016/j.compchemeng.2020.107004.
- Müller, Ch., Diedam, H. Mrziglod, Th., Schuppert A., (2020) A neural network assisted Metropolis adjusted Langevin algorithm, Monte Carlo Methods and Applications, Volume 26: Issue 2, https://doi.org/10.1515/mcma-2020-2060.
- N.Kusch, A. Schuppert (2020), Two-step multi-omics modelling of drug sensitivity in cancer cell lines to identify driving mechanisms, PLoS One, doi.org/10.1371/journal.pone.0238961.
- Malvin Jefri, Scott Bell, Huashan Peng, Nuwan Hettige, Gilles Maussion, Vincent Soubannier, Hanrong Wu, Heika `Silveira, Jean-Francoix Theroux, Luc Moquin, Xin Zhang, Zahia Aouabed, Jeyashree Krishnan, Liam A. O’Leary, Lilit Antonyan, Ying Zhang, Vincent McCarty, Naquib Mechawar, Alain Gratton, Andreas Schuppert, Thomas M. Durcan, Edward A. Fon, Carl Ernst (2020) Stimulation of L‐type calcium channels increases tyrosine hydroxylase and dopamine in ventral midbrain cells induced from somatic cells, Stem Cells Translational Medicine, https://doi.org/10.1002/sctm.18-0180.
- Schuppert, A., Theisen, S., Fränkel, P., Weber-Carstens, S., Karagiannidis, C. (2021) Bundesweites Belastungsmodell für Intensivstationen durch COVID-19, Medizinische Klinik – Intensiv- und Notfallmedizin, https://link.springer.com/article/10.1007/s00063-021-00791-7#auth-A_-Schuppert, doi.org/10.1007/s00063-021-00791-7.
- Schuppert, A., Polotzek, K., Schmitt,J., Busse,R., Karschau, J., Karagiannidis, Ch. (2021) Different spreading dynamics throughout Germany during the second wave of the COVID-19 pandemic: a time series study based on national surveillance data, The Lancet Regional Health Europe, 26. June 2021, DOI: doi.org/10.1016/j.lanepe.2021.100151DOI.
- Peine, A., Hallawa, A., Bickenbach, J., Dartmann G., Begic Fazlic, L., Schmeink, A., Ascheid, G., Thiemermann, C., Schuppert, A., Kindle, R., Celi, L., Marx, G., Martin L. Development and validation of a reinforcement learning algorithm to dynamically optimize mechanical ventilation in critical care. npj Digit. Med. 4, 32 (2021). https://doi.org/10.1038/s41746-021-00388-6.
- Günster, Ch., Busse, R., Spoden, M., Rombey, T., Schillinger, G., Hoffmann, W., Weber-Carstens, S., Schuppert, A., Karagiannidis, Ch. (2021) 6-month mortality and readmissions of hospitalized COVID-19 patients: A nationwide cohort study on 8679 patients in Germany, PLoS One, https://doi.org/10.1371/journal.pone.0255427.
- Schuppert, A., Weber-Carstens, S. & Karagiannidis, C. Intensivbettenbedarf für COVID‑19 im Herbst/Winter 2021. Med Klin Intensivmed Notfmed (2021). https://doi.org/10.1007/s00063-021-00862-9.
- Ali Hadizadeh Esfahani, Janina Maß, Asis Hallab, Bernhard M Schuldt, David Nevarez, Björn Usadel, Mark-Christoph Ott, Benjamin Buer, Andreas Schuppert, Plant PhysioSpace: a robust tool to compare stress response across plant species, Plant Physiology, 2021;, kiab325, https://doi.org/10.1093/plphys/kiab325.
- Baumeister, J., Maié, T., Chatain, N., Gan, L., Weinbergerova, B., de Toledo M., Eschweiler, J., Maurer A., Mayer J., Kubesova B., Racil Z., Schuppert, A., Costa I., Koschmieder S., Brümmendorf T.H., Gezer D.Early and late stage MPN patients show distinct gene expression profiles in CD34+ cells. Ann Hematol (2021). https://doi.org/10.1007/s00277-021-04615-8.
- Scott Bell, Vincent McCarty, Huashan Peng, Malvin Jefri, Nuwan Hettige, Lilit Antonyan, Liam Crapper, Liam A. O'Leary, Xin Zhang, Ying Zhang, Hanrong Wu, Diane Sutcliffe, Ilaria Kolobova, Thad A. Rosenberger, Luc Moquin, Alain Gratton, Jelena Popic, Ilse Gantois, Patrick S. Stumpf, Andreas A. Schuppert, Naguib Mechawar, Nahum Sonenberg, Michel L. Tremblay, Hyder A. Jinnah, Carl Ernst, Lesch-Nyhan disease causes impaired energy metabolism and reduced developmental potential in midbrain dopaminergic cells, Stem Cell Reports, Volume 16, Issue 7, 2021, Pages 1749-1762, ISSN 2213-6711, https://doi.org/10.1016/j.stemcr.2021.06.003.
- Oliver Maassen, MSc; Sebastian Fritsch, MD; Julia Palm, MSc; Saskia Deffge, MSc; Julian Kunze, MD; Gernot Marx, MD, Prof Dr, FRCA; Morris Riedel, Prof Dr; Andreas Schuppert, Prof Dr; Johannes Bickenbach, MD, Prof Dr (2021) Future Medical Artificial Intelligence Application Requirements and Expectations of Physicians in German University Hospitals: Web-Based Survey , Journal of Medical Internet Research.
- Schuppert, Andreas and Polotzek, Katja and Karschau, Jens and Karagiannidis, Christian, (2021) Effectiveness of extended shutdown measures during the ´Bundesnotbremse´introduced in the third SARS-CoV-2 wave in Germany,Infection,https://doi.org/10.1007/s15010-021-01713-7.
- Claudia Merger, Timo Reinartz, Stefan Wessel, Carsten Honerkamp, Andreas Schuppert, Moritz Helias, Global hierarchy vs. local structure: spurious self-feedback in scale-free networks, Phys. Rev. Research 3.033272.
- Sebastian Fritsch, Konstantin Sharafutdinov, Gernot Marx, Andreas Schuppert, Johannes Bickenbach (2021) Biometric covariates and outcome in COVID-19 patients: Are we looking close enough?, BMC Infect Dis. 2021 Nov 4;21(1):1136. doi: 10.1186/s12879-021-06823-z.
- Kilian Merkelbach, Artur M. Schweidtmann, Younes Müller, Patrick Schwoebel, Adel Mhamdi, Alexander Mitsos, Andreas Schuppert, Thomas Mrziglod, Sebastian Schneckener, HybridML: Open source platform for hybrid modeling, Computers & Chemical Engineering, Volume 160, 2022, 107736,ISSN 0098-1354, https://doi.org/10.1016/j.compchemeng.2022.107736.
- Fritsch S, Sharafutdinov K, Schuppert A, Bickenbach J. Nutzung von künstlicher Intelligenz zur Bekämpfung der COVID-19-Pandemie [Usage of Artificial Intelligence in the Combat against the COVID-19 Pandemic]. Anasthesiol Intensivmed Notfallmed Schmerzther. 2022 Mar;57(3):185-197. German. doi: 10.1055/a-1423-8039. Epub 2022 Mar 23. PMID: 35320841.
- Hettige NC, Peng H, Wu H, Zhang X, Yerko V, Zhang Y, Jefri M, Soubannier V, Maussion G, Alsuwaidi S, Ni A, Rocha C, Krishnan J, McCarty V, Antonyan L, Schuppert A, Turecki G, Fon EA, Durcan TM, Ernst C. FOXG1 dose tunes cell proliferation dynamics in human forebrain progenitor cells. Stem Cell Reports. 2022 Mar 8;17(3):475-488. doi: 10.1016/j.stemcr.2022.01.010. Epub 2022 Feb 10. PMID: 35148845; PMCID: PMC9040178.
- Schuppert A, Karagiannidis C. Dynamische Entwicklung der COVID-19-Intensivbelegung im Herbst/Winter 2021/22 in Abhängigkeit von den 7-Tage-Inzidenzen [Dynamic simulation of COVID-19 intensive care bed occupancy in fall/winter 2021/22 as a function of 7-day incidences]. Med Klin Intensivmed Notfmed. 2022 Apr;117(3):206-208. German. doi: 10.1007/s00063-022-00904-w. Epub 2022 Feb 9. PMID: 35138413; PMCID: PMC8983622.
- Stalmann USA, Ticconi F, Snoeren IAM, Li R, Gleitz HFE, Cowley GS, McConkey ME, Wong AB, Schmitz S, Fuchs SNR, Sood S, Leimkühler NB, Martinez-Høyer S, Banjanin B, Root D, Brümmendorf TH, Pearce JE, Schuppert A, Bindels EMJ, Essers MA, Heckl D, Stiehl T, Costa IG, Ebert BL, Schneider RK. Genetic barcoding systematically compares genes in del(5q) MDS and reveals a central role for CSNK1A1 in clonal expansion. Blood Adv. 2022 Mar 22;6(6):1780-1796. doi: 10.1182/bloodadvances.2021006061. PMID: 35016204; PMCID: PMC8941465.
- Jefri M, Zhang X, Stumpf PS, Zhang L, Peng H, Hettige N, Theroux JF, Aouabed Z, Wilson K, Deshmukh S, Antonyan L, Ni A, Alsuwaidi S, Zhang Y, Jabado N, Garcia BA, Schuppert A, Bjornsson HT, Ernst C. Kabuki syndrome stem cell models reveal locus specificity of histone methyltransferase 2D (KMT2D/MLL4). Hum Mol Genet. 2022 May 28:ddac121. doi: 10.1093/hmg/ddac121. Epub ahead of print. PMID: 35640156.
- Saldanha OL, Quirke P, West NP, James JA, Loughrey MB, Grabsch HI, Salto-Tellez M, Alwers E, Cifci D, Ghaffari Laleh N, Seibel T, Gray R, Hutchins GGA, Brenner H, van Treeck M, Yuan T, Brinker TJ, Chang-Claude J, Khader F, Schuppert A, Luedde T, Trautwein C, Muti HS, Foersch S, Hoffmeister M, Truhn D, Kather JN. Swarm learning for decentralized artificial intelligence in cancer histopathology. Nat Med. 2022 Jun;28(6):1232-1239. doi: 10.1038/s41591-022-01768-5. Epub 2022 Apr 25. PMID: 35469069; PMCID: PMC9205774.
- E. Samadi M, Kiefer S, Fritsch SJ, Bickenbach J, Schuppert A (2022) A training strategy for hybrid models to break the curse of dimensionality. PLOS ONE 17(9): e0274569. doi.org/10.1371/journal.pone.0274569
- Sharafutdinov K, Bhat JS, Fritsch SJ, Nikulina K, E Samadi M, Polzin R, Mayer H, Marx G, Bickenbach J, Schuppert A. Application of convex hull analysis for the evaluation of data heterogeneity between patient populations of different origin and implications of hospital bias in downstream machine-learning-based data processing: A comparison of 4 critical-care patient datasets. Front Big Data. 2022 Oct 31;5:603429. doi: 10.3389/fdata.2022.603429. PMID: 36387013; PMCID: PMC9659720.
- K. Sharafutdinov et al., "Computational simulation of virtual patients reduces dataset bias and improves machine learning-based detection of ARDS from noisy heterogeneous ICU datasets," in IEEE Open Journal of Engineering in Medicine and Biology, doi: 10.1109/OJEMB.2023.3243190.
- Merkelbach K, Schaper S, Diedrich C, Fritsch SJ, Schuppert A. Novel architecture for gated recurrent unit autoencoder trained on time series from electronic health records enables detection of ICU patient subgroups. Sci Rep. 2023 Mar 11;13(1):4053. doi: 10.1038/s41598-023-30986-1. PMID: 36906642; PMCID: PMC10008580.
- Egwu, N., Mrziglod, T. & Schuppert, A. Neural network input feature selection using structured l2 − norm penalization. Appl Intell 53, 5732–5749 (2023). doi.org/10.1007/s10489-022-03539-8
- Samadi ME, Guzman-Maldonado J, Nikulina K, Mirzaieazar H, Sharafutdinov K, Fritsch SJ, Schuppert A. A Hybrid Modeling Framework for Generalizable and Interpretable Predictions of ICU Mortality: Leveraging ICD Codes in a Multi-Hospital Study of Mechanically Ventilated Influenza Patients, https://doi.org/10.21203/rs.3.rs-3236927/v1
Barakat, C.; Aach, M.; Schuppert, A.; Brynjólfsson, S.; Fritsch, S.; Riedel, M. Analysis of Chest X-ray for COVID-19 Diagnosis as a Use Case for an HPC-Enabled Data Analysis and Machine Learning Platform for Medical Diagnosis Support. Diagnostics 2023, 13, 391. https://doi.org/10.3390/diagnostics13030391
Nuwan C Hettige, Peter Fleming, Amelia Semenak, Xin Zhang, Huashan Peng, Marc-Daniel Hagel, Jean-François Théroux, Ying Zhang, Anjie Ni, Malvin Jefri, Lilit Antonyan, Shaima Alsuwaidi, Andreas Schuppert, Patrick S Stumpf, Carl Ernst, FOXG1 targets BMP repressors and cell cycle inhibitors in human neural progenitor cells, Human Molecular Genetics, Volume 32, Issue 15, 1 August 2023, Pages 2511–2522, https://doi.org/10.1093/hmg/ddad089
Barakat CS, Sharafutdinov K, Busch J, Saffaran S, Bates DG, Hardman JG, Schuppert A, Brynjólfsson S, Fritsch S, Riedel M. Developing an Artificial Intelligence-Based Representation of a Virtual Patient Model for Real-Time Diagnosis of Acute Respiratory Distress Syndrome. Diagnostics. 2023 Jun 17;13(12):2098.
Polzin R, Fritsch S, Sharafutdinov K, Marx G, Schuppert A. Diagnostic Expert Advisor: A platform for developing machine learning models on medical time-series data. SoftwareX. 2023 Jul 1;23:101517.
B. Patents
- A.Schuppert (1980): Verfahren und Vorrichtung zur zweidimensionalen stroboskopischen Abtastung der Lineargeschwindigkeit eines Körpers. German Patent no. P2833262.9.
- B.Lohmann, A.Schuppert, J.Kämpfer, M.Warncke (2001) Method for determining a complex correlation pattern from process and plant data (EP 1313030).
- A.Schuppert (2001): Method for the identification of pharmacophores (EP 1451750).
- A.Schuppert, A.Ohrenberg (2003): Method and computer for experimental design (PCT/EP03/03424 (WO)).
- A.Schuppert, R.Burghaus, C.v.Törne, S.Schwers, U.Strop, H.Kallabis (2007): Method for identifying predictive biomarkers from patient data, WO/2007/07/9875.
- A.Schuppert, H.Ellinger-Ziegelbauer, H.J.Ahr (2008): Method for determining the behavior of a biological system after a reversible disturbance, WO/2008/006469.